What artificial intelligence can teach us about the air we breathe – and what we have to teach it
Changes in our atmosphere, driven by societal emissions, have begun to affect our daily existence. Air polluted with ozone and particulate matter is linked to the deaths of millions of people a year. Increased temperatures caused by global climate change have led to many more public health risks, including increasingly severe wildfires, which emit vast amounts of particulate matter that is transported by the wind across state and international borders. These linked environmental issues drive a system of complex interactions on a regional and global scale. Understanding these interactions is crucial for scientifically informed environmental policy. Computer simulations of atmospheric processes help us predict future environmental effects of our present-day societal decisions.
At the same time, artificial intelligence or AI, a set of algorithms that learn patterns from data, has started to creep into our everyday lives. Recommender systems control social media feeds, images can be generated from text prompts, and large language models like ChatGPT can help us write convincing emails, articles, and blog posts. Though these are exciting new developments, one challenge of AI models like ChatGPT is knowing when to trust them. Even when the data doesn’t lie, the data-driven algorithm can.
My name is Obin Sturm, and I’m a 2023 Sonosky Fellow working at the intersection of air quality and AI in the Earth Data Science and Atmospheric Composition group led by Professor Sam Silva. My research focuses on how we can use AI and other data-driven approaches to improve air quality and climate models. I think it’s very important to ensure these data-driven algorithms deliver scientifically trustworthy results.
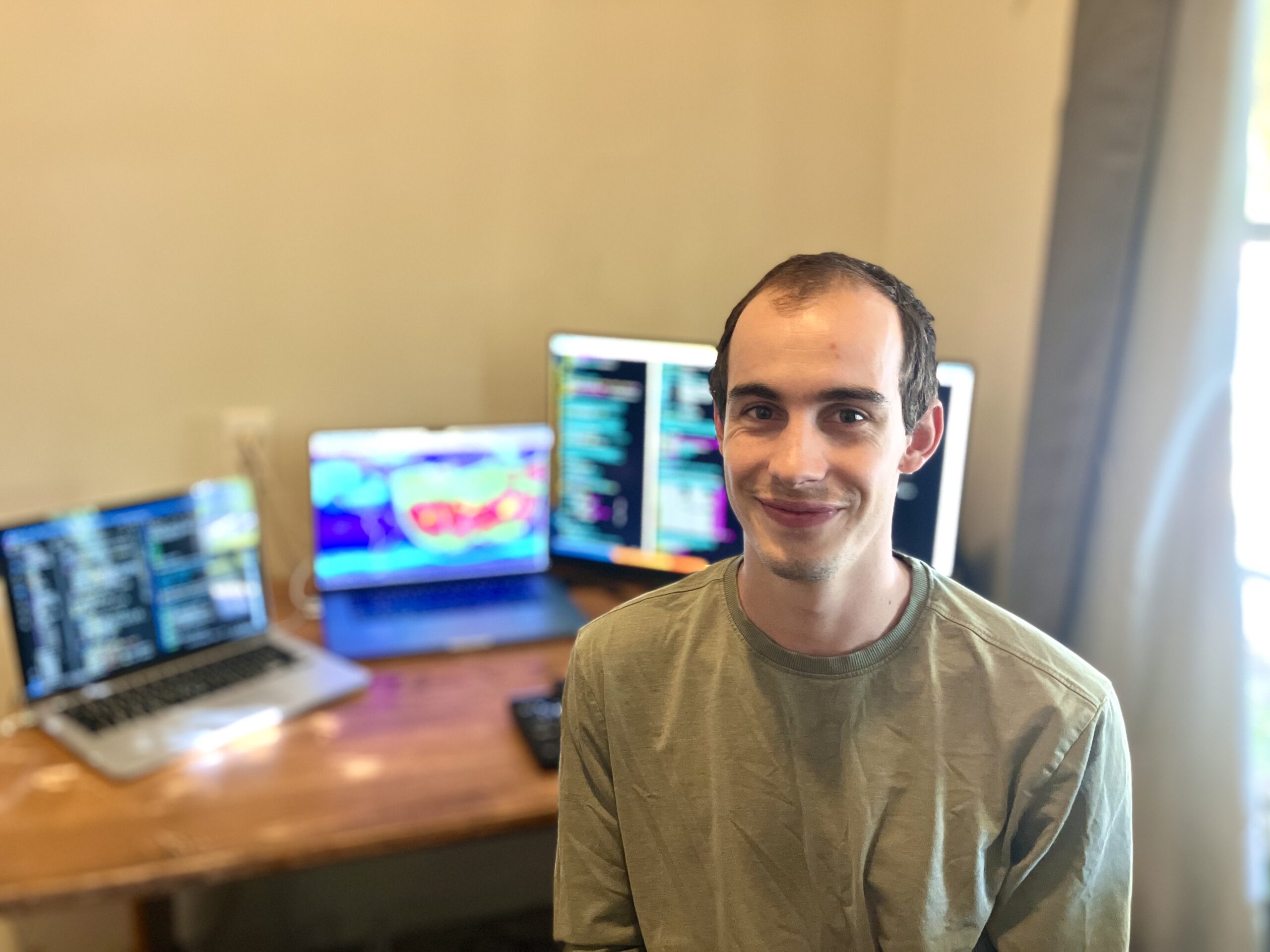
How can AI improve atmospheric simulations? What can it teach us?
Some data-driven algorithms are good at finding simple patterns in complex data. This enables more realistic yet efficient simulations of complex processes. One project I worked on (published this spring in Journal of Advances in Modeling Earth Systems for a special issue on machine learning!) found patterns in the concentrations of secondary organic aerosol, which is an important component of particulate pollution. This allows a simple representation of particulate matter for wind transport but full complexity for aerosol formation, enabling a more realistic representation of particulate pollution in air quality forecasts for Europe.
This summer as a Sonosky Fellow, I am continuing to study air pollution with data-driven techniques. Given only data on pollutant concentrations, can we use AI to infer the complex network of chemical reactions behind the scenes? This knowledge is essential for accurate air quality models and is usually obtained in a lab one reaction at a time. I am hoping to develop a complementary approach by building on an existing technique called a chemical reaction neural network and applying it first to a simple smog model. So far, the technique is able to rediscover one known reaction: that ozone reacts with nitric oxide, a primary pollutant from car exhaust. However, this is just one of 10 reactions in the reaction network, and one of many more in the actual atmosphere, so we have a long way to go. Chemistry can be hard to learn, even for AI!
Many atmospheric models, like the smog model I’m trying to teach to AI, are not data-driven but rather based on our understanding of how the universe works. Such models often obey fundamental physical laws, for example mass conservation: “matter cannot be created nor destroyed.” A second part of my summer fellowship research, in collaboration with researchers at the Tegmark group at MIT, tested a data-driven tool that is designed to discover invariants from data, such as mass conservation during chemical reactions.
We analyzed simulated concentrations from the smog model with the hypothesis that this data-driven technique would find the built-in carbon and nitrogen conservation. However, we not only rediscovered both conservation laws, but also a third unexpected constant. This third conserved quantity is a combination of several pollutant concentrations that stays constant over a wide variety of atmospheric conditions, even when the chemistry is changing rapidly. Earlier this summer, we submitted some of this work to a journal for peer-review (arXiv preprint), and it also got some attention on Twitter (see below).
With our new #AI algorithm, we finally managed to discover new conservation laws that domain experts didn’t know about, in both fluid mechanics and atmospheric chemistry: https://t.co/yWjNtkE2O3 pic.twitter.com/0vtEcp7Kx1
— Max Tegmark (@tegmark) June 1, 2023
We have yet to determine if this third conserved quantity is just a limitation of the computational simulation or has a real-world meaning. Personally, I’d find either result quite interesting! It is so exciting that data-driven techniques can teach us something new about a system we thought we understood well.
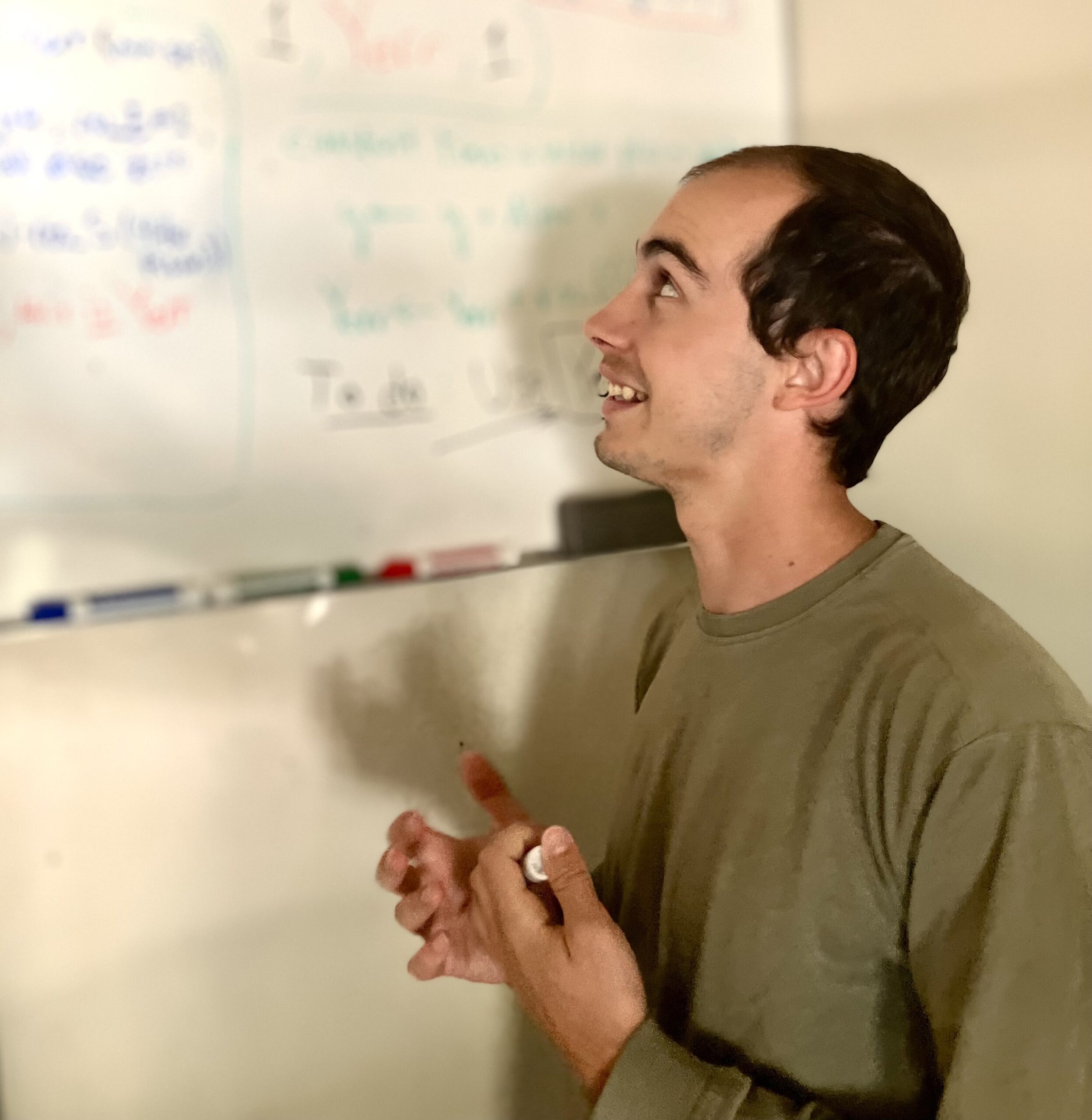
How do we teach AI to be trustworthy, from a scientific perspective?
Remember the concern that ChatGPT can sometimes give false information? Some AI models of atmospheric processes have the same issue: a purely data-driven model learns from data without understanding the fundamental processes behind the data. Such a model can produce output that violates conservation laws, leading to results that don’t make scientific sense and can’t be trusted.
To address this issue, a new branch of AI called physics-informed machine learning hybridizes AI techniques with scientific fundamentals. In 2020, Professor Anthony Wexler at UC Davis and I published a mass- and energy- conserving framework for machine learning for geoscientific simulations, now integrated into AI algorithms for smog chemistry as well as particulate matter over the Amazon rainforest. For atmospheric chemistry, this framework is the known reaction network which inherently conserves mass. My research supported by the Sonosky Fellowship builds off of this, aiming to discover unknown reaction networks from data alone! AI algorithms struggle to learn atmospheric chemistry, but they get a lot better when we teach them what we already know. Why make them start from scratch?
Finally, access to good data is important for any AI technique to function well. I am collaborating with the NASA Global Modeling and Assimilation Office to put together a detailed dataset, powered by the Earth system model GEOS-CF that provides global forecasts of the chemical composition of the atmosphere. This dataset contains high-quality estimates of nearly 300 chemical species and reaction rates over four seasons and a wide variety of atmospheric conditions – from rainforests to deserts, dense cities to the middle of the oceans. We’re using this dataset to explore patterns in twilight chemistry. Twilight chemistry is interesting to study because some of the quickest chemical changes happen during sunrise and sunset: this also makes twilight chemistry one of the computational bottlenecks in global forecasting. The GEOS-CF dataset will also support projects in our research group involving physics-informed machine learning and reaction networks. This includes a particularly exciting project on chemical cycles and network analysis led by Emy Li, an undergraduate researcher in our group who I am collaborating with.
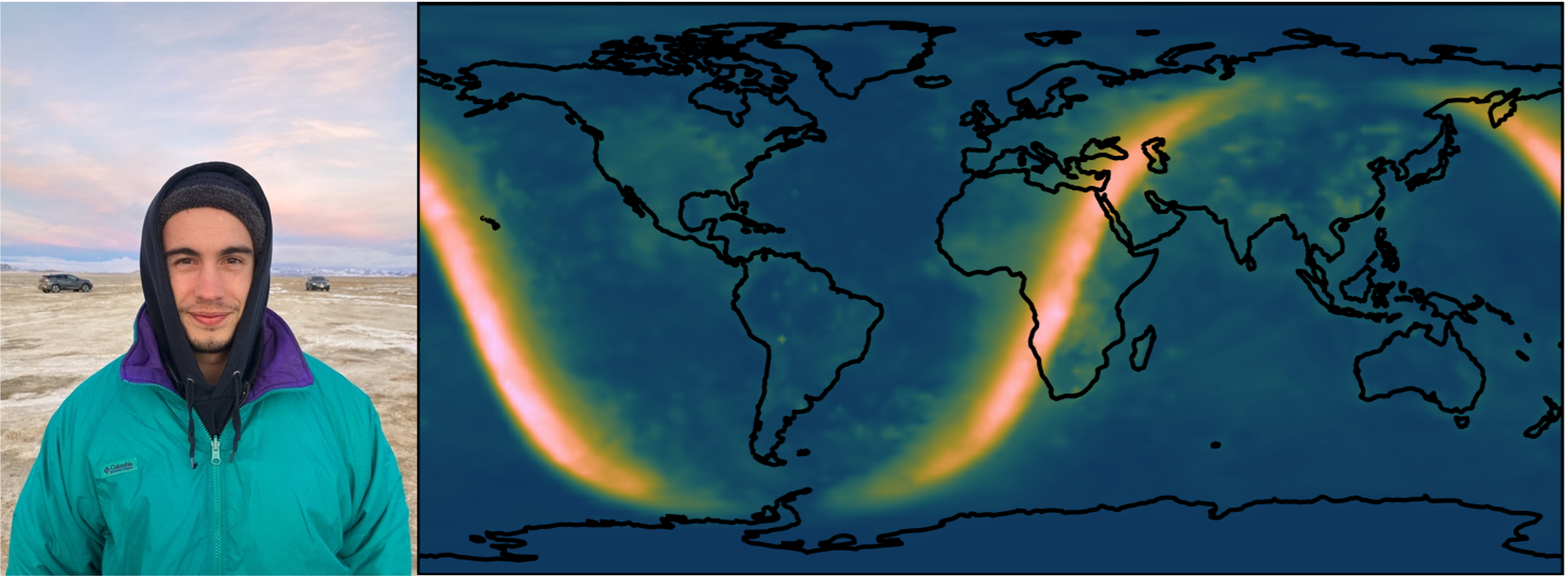
AI has the potential to help us understand the complex Earth system to better address urgent environmental challenges like air pollution and climate change; AI will be even more useful if it has our prior scientific discoveries and knowledge built into it, inherently. The dawn of hybridizing scientific knowledge and AI illuminates a path towards a deeper understanding of our world from data, which can support scientifically informed environmental policies for cleaner air and sustainable policies – an inspiring journey to be a part of!
Obin Sturm is supported by the Diane Sonosky Montgomery and Jerol Sonosky Graduate Fellowship for Environmental Sustainability Research. Read Obin’s published paper here.